Explainable AI and Mātauranga Māori For Diagnosis and Prognosis in Mental Health
The workshop aims to foster a 3-hour discussion on recent progress and challenges in the early prediction of mental and neurological health outcomes using Explainable AI techniques and multimodal sets of data. The workshop will also discuss on Mātauranga Māori and Artificial intelligence: “hinengaro” a vision for the future.
Opening: The workshop will start and conclude by our esteemed Māori collaborators.
- New Zealand Singapore Data Science Research program, Computational Neuro-genetic modelling for mental health, Demonstration of NeuCube Software by Dr Maryam Doborjeh, Auckland University of Technology, New Zealand.
- Longitudinal Data Collection in Mental Health by Dr Jimmy Lee, Institute of Mental Health, Singapore.
- The Utility of Text Analysis and Sentiment Analysis for Mental Health Prediction by Asst Prof Wilson Goh, Nanyang Technological University, Singapore.
- Predictive Modelling on Integrated Longitudinal Cognitive, Clinical and Genetic Data for Early Individual Risk of Psychosis Prediction by Dr Zohreh Doborjeh, The University of Auckland, New Zealand.
- Multimodal learning on biomedical dataset by Balkaran Singh and Professor Edmund Lai, Auckland University of Technology, New Zealand
- TBA by S. Tan, Nanyang Technological University, Singapore.
- Sugam Budhraja, Auckland University of Technology, New Zealand.
- Software demonstration on Personalised Predictive Modelling on Multimodal Data (PPMMD), a tool developed by the joint Singapore-New Zealand team. Presenters: Sugam Budhraja, Balkaran Singh, Samuel Tan.
- Maintaining Mātauranga Māori in AI and health research and sustainability by Dr Pouroto Ngaropo, Ms. Sandra Potaka and Dr Margaret Hinepo Williams.
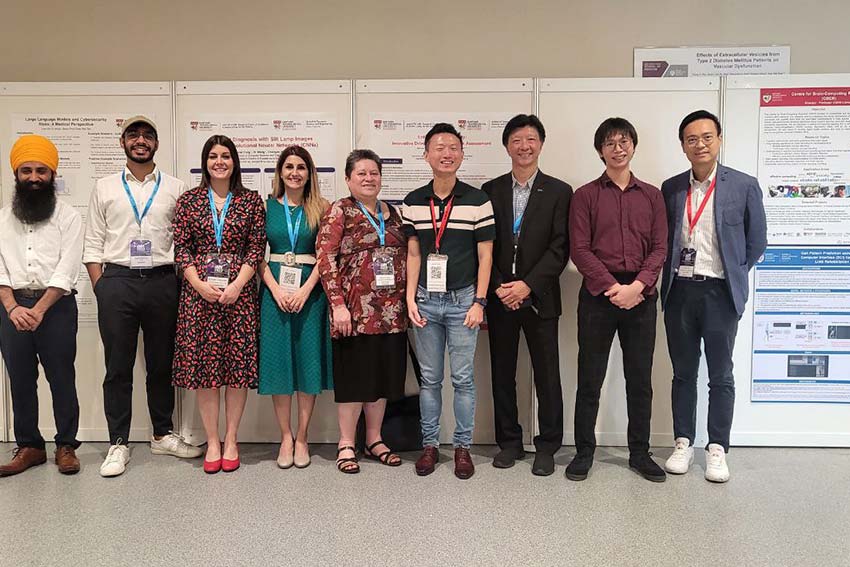
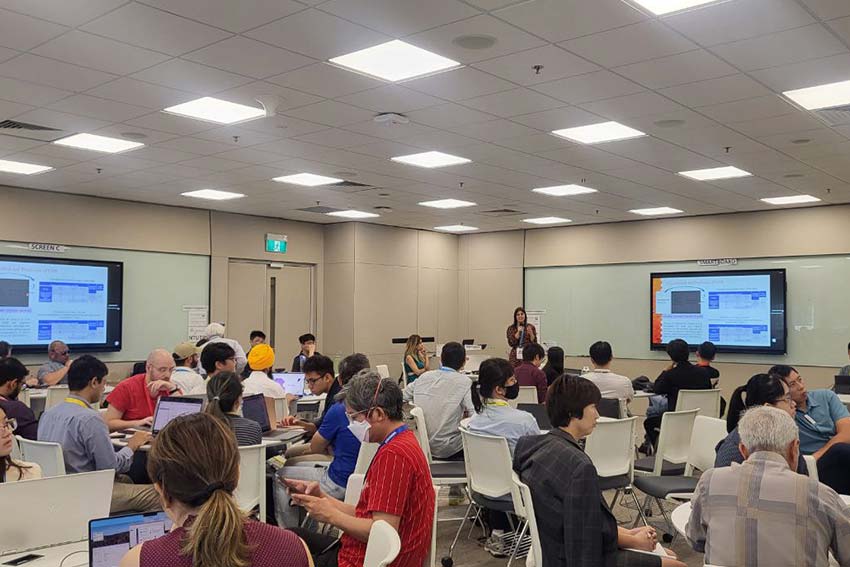
Closing Mihi.
Workshop description
This workshop is based on two main scope that integrate science and technology with Indigenous values and knowledge towards developing and applying AI for improving health and wellbeing.
Explainable AI for diagnosis and prognosis in mental health
Mental illnesses have risen in ranking as a leading cause of DALYs (Disability-adjusted Life Years) from 13 in 1990 to 7 in 2017. About 75% of mental illnesses have their onset before the age of twenty-five and cause chronic disability, making mental illnesses the second leading cause of years lived with disability (YLD). Accurate prediction of an individual's risk of mental illness development requires advanced computational techniques to model multimodal sets of data, including spatiotemporal brain data, cognitive assessments, and genetic and molecular data. Traditional artificial intelligence and machine learning (AI/ML) models, such as deep convolutional neural networks (CNNs), are inadequate as they lack interpretability and cannot capture temporal effects. This limits their ability to generate knowledge from data, and they also function as black boxes that offer limited insight into their decision-making processes. Therefore, the workshop aims to develop advanced AI and machine learning algorithms that improve both model accuracy and explainability.
Mātauranga Māori and artificial intelligence: “hinengaro” a vision for the future
A comprehensive workshop is designed to weave Mātauranga Māori (Māori values, knowledge, practices, and experiences) and artificial intelligence paradigms to focus on the future optimal wellbeing of vulnerable populations. The example is to improve health outcomes for Māori, the indigenous people of Aotearoa. Three key components will be discussed: i. the kairangahau (researcher) as the interface to connect the research team and vision matauranga stakeholders; ii. creating a space for korero (discussion) about utilising artificial intelligence to improve health outcomes; and iii to better understand Hinengaro. To better understand Hinengaro we will explore the concepts of wairua (spirituality), mauri (life force), and toiora (optimal health and wellbeing). Typically, mātauranga Māori encompasses three levels of trajectory i). original, ii) traditional and iii) contemporary. In each trajectory timeline Māori have had the agility and capacity to innovate and respond to the challenges faced. Māori continue to embrace new technologies and so artificial intelligence provides a platform for their future.
The workshop seeks to bring together researchers from the fields of AI, machine learning, bioinformatics, and Neuroinformatics to present the state-of-the-art in the development of interpretable and expandable computational models for the diagnosis and prognosis of mental and neurological health outcomes.
Organising committee
The organising committee comprises an interdisciplinary research team formed through a cross-discipline, cross-university, and international collaboration. The team combines expertise from information technology, AI, biomedical sciences, psychology, neuroscience, psychiatry and mātauranga Māori to address the issue of modelling high-dimensional data sets for the diagnosis and prognosis of mental health outcomes. The team includes early career researchers, experts from Māori health, education, and language along with senior researchers.
- Dr Maryam Doborjeh, Senior Lecturer, AUT, Aotearoa New Zealand
- Dr Zohreh Doborjeh, The University of Auckland, Aotearoa New Zealand
- PhD students: Sugam Budhraja (AUT, Aotearoa New Zealand), Balkaran Singh (AUT, Aotearoa New Zealand), Samuel Tan (NTU, Singapore)
- Assistant Professor Wilson Goh, Biomedical Informatics, Lee Kong Chian School of Medicine Nanyang Technological University, Singapore
- Professor Nikola Kasabov, Life FIEEE, FRSNZ, FINNS, AUT, Aotearoa New Zealand
- Professor Edmund Lai, AUT, Aotearoa New Zealand
- Dr Jimmy Lee, Institute for Mental Health, Singapore
- Dr Pouroto Ngaropo, Iramoko Marae, Te Tarewa Hapu o Ngāti Awa, Aotearoa New Zealand
- Ms Sandra Potaka, Wairakei Māori Women’s Welfare League (MWWL), Waiariki, Aotearoa New Zealand
- Dr Margaret Hinepo Williams, AUT, Auckland, Aotearoa New Zealand
References
[1] Zohreh Doborjeh, Maryam Doborjeh, Alexander Sumich, Balkaran Singh, Alexander Merkin, Sugam Budhraja, Wilson Wen Bin Goh, Edmund Lai, Margaret Williams, Samuel Tan, Jimmy Lee, and Nikola Kasabov, Investigation of Social and Cognitive Predictors in Non-Transition Ultra-High-Risk’ Individuals for Psychosis Using Spiking Neural Networks, Schizophrenia, 9, 10 (2023), https://doi.org/10.1038/s41537-023-00335-2.
[2] Balkaran Singh, Maryam Doborjeh, Zohreh Doborjeh, Sugam Budhraja, Samuel Tan, Alexander Sumich, Wilson Goh, Jimmy Lee, Edmund Lai, Nikola Kasabov, Constrained Neuro Fuzzy Inference Methodology for Explainable Personalised Modelling with Applications on Gene Expression Data, Scientific Reports, 13-456, 2023, https://www.nature.com/articles/s41598-022-27132-8, Nature Publ., 2023.
[3] N. Kasabov, Yongyao Tan; Doborjeh, Maryam; Tu, Enmei; Yang, Jie (2023): Transfer Learning of Fuzzy Spatio-Temporal Rules in the NeuCube Brain-Inspired Spiking Neural Network: A Case Study on EEG Spatio-temporal Data. TechRxiv. Preprint. https://techrxiv.org), https://doi.org/10.36227/techrxiv.21781103.v1.
[4] S. Budhraja, B. Singh, S. Tan, M. Doborjeh, Z. Doborjeh, W. Goh, E. Lai, and N. Kasabov, Mosaic LSM: A Liquid State Machine Approach for Multimodal Longitudinal Data Analysis), 2023 International Joint Conference on Neural Networks (IJCNN), Australia, 18-25 June 2023.
[5] NeuCom platform: https://theneucom.com
[6] NeuCube platform: https://kedri.aut.ac.nz/neucube